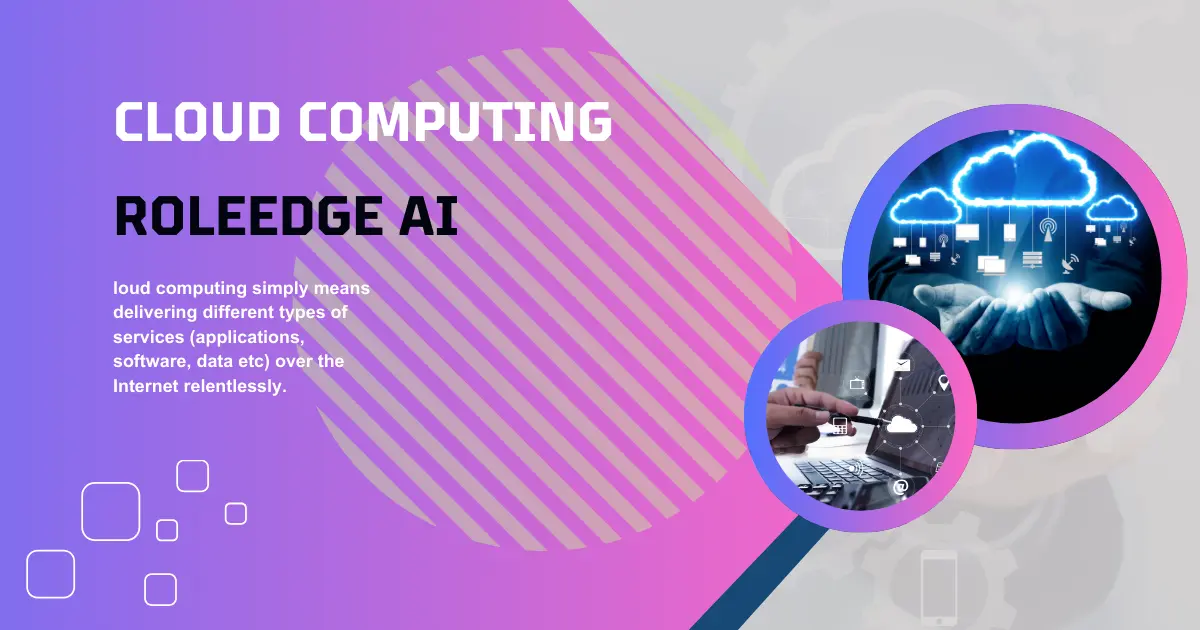
Cloud Computing and the Roleedge AI
The Revolution in Data Management & Processing with Cloud computing: Cloud Computing has become indispensable today and is defined as the delivery of scalable resources through Internet. However, data is growing exponentially so the demand for on-the-spot processing and low-latency responses has fostered edge AI. In this piece, we delve deeper into cloud computing and edge AI to see how they intersect with one another in order – combing their features together to better our digital experiences.
What is Cloud Computing?
Cloud computing simply means delivering different types of services (applications, software, data etc) over the Internet relentlessly. It provides the following service types:
- IaaS: Typically, Iaas is a virtualized resource offering over the internet.
- Platform as a Service (PaaS): Delivering a computing platform and/or solution stack as an integrated service, allows developers to develop software applications without the cost and complexity of buying & managing hardware,software or stacked management middleware.
- Software as a Service (SaaS): Examples of these include Software as a Service (SaaS) and provide software applications over the internet on request, whilst charging Monthly or Subscription based fees.
Cloud computing has many advantages, such as cost efficiencies, scalability and flexibility in addition to better collaboration. Traditional cloud computing can lag in reading or processing data files quickly for real-time analysis as it grows and becomes more complex.
Understanding Edge AI
Edge AI is like a new quantum leap in data handling and processing. This entails deploying AI algorithms closer to the edge of a network, on local devices where data is actually produced. Edge computing allows data processing to occur locally, instead of in centralized data centers hundreds or thousands of miles away saving time and reducing the amount bandwidth consumed than traditional cloud computing.
The Edge AI backbone comprises potent edge devices, sophisticated AI algorithms, and distributed computing frameworks. The fusion of these disciplines has made possible the process data more quickly, analytics in real time and to even through regions with little coverage.
The Role of Edge AI in Cloud Computing
Edge AI complements cloud computing through removing data processing jobs from central servers as well as shifting them to edge gadgets.
- Improved Data Processing and Analytics: Edge AI helps to process data much closer to the source of data helping reduce the amount of explosion in terms of volume that gets transmitted over network leading faster analysis.
- Latency and Performance Tunings: Analyzing data in real time at the edge improves response times for applications such as autonomous vehicles or industrial automation.
- This enables the capabilities of real-time decision-making: That is important in applications such as Healthcare monitoring or financial trading where milliseconds matter.
Key Characteristics of Cloud Computing.
What It Means to Merge Edge AI with Cloud Computing
The following are some of the advantages offered by edge AI enabled cloud computing :
- Scalability and Flexibility- Businesses can confidently scale their operations through edge and cloud resources, providing the best performance for any workload.
- Better Data Security and Privacy: By processing data at the edge location, this information can be stored on-premise away from potential breaches through Internet transmission.
- Budget-Friendly and Resource Optimized: When it comes to budget, Edge AI is a savior allowing you to minimize data transfers on the cloud by retaining useful decoded information on premises thus, ultimately reducing both bandwidth as well as cost chats associated with incurring unnecessary redundant writing of transactions which would eventually make your pockets dry.
Edge AI Cloud Computing Use Cases
There are numerous industries and sectors in which edge AI can be employed, including:
- Edge AI for Smart Cities and IoT Applications: In all from traffic management to environmental monitoring, then what is the role of edge 1?
- Healthcare and Remote Monitoring: Edge AI is able to keep up-to-date with the overall monitor of health conditions in real time which can help on demanding systems – such as a data-driven centralized healthcare system.
- Autonomous Vehicles and Transportation: Up to the second data processing is critical for navigation, safety, decision making in autonomous vehicles.
- IIoT Edge for Industrial Automation and Predictive Maintenance: With the help of Edge AI, device can keep an eye on it self as well machinery and predict when maintenance is needed beforehand so that production never has to stop.
Challenges and Considerations
Although edge AI in cloud technology provides many advantages, the method has its own limitations:
- Data Security and Privacy Concerns: This platform introduces new vulnerabilities and complexity in data privacy while at the same reducing complexities related to security.
- Network Reliability and Bandwidth Problems: since networks are crucial for the operation of edge computing, they often become unreliable or get low bandwidth which can be difficult in some remote areas bit
- Deployment and management complexity: Deploying, as well as managing edge AI systems can be exceedingly complex due to the specialized skills, which are needed both during setup time of an environment that a model is able to run in efficiently.
CONCLUSION | Future Of Cloud Computing And Machine Learning(Expression AI)
As we move forward, there are a few key trends that promise to drive the future of cloud computing and edge AI:
- How 5G Drives Edge AI: The infusion of 5G technology will increase the potential for edge computing by allowing faster communication between devices leading to reduced latency.
- Continued AI and Machine Learning Innovations: Continued digital innovation will enhance edge artificial intelligence (AI) / machine learning tasks which in turn makes them more accurate and efficient.
- Hybrid and Multi-Cloud: Balancing Cloud with Edge – As many are starting to see, it is beneficial for organizations that are embracing both cloud platforms as well as edge computing.
Edge AI and cloud computing are two very different technologies making this story even more compelling. With data constantly increasing in both volume and velocity, more SaaS providers understand edge AI is the only way to compete against rivals that are now able to add cloud services on top of their existing products. Greater efficiency, security and innovation are realized by using the best of both.
FAQs
While we have discussed cloud computing at length in this article, the biggest difference lies between cloud computing and edge computing?
This way cloud computing focuses on data processing from centralized locations such as remote data centers, but the edge fits in to process of storing and moving this data closer towards its source thus minimizing latency time as well amount of bandwidth used.
The edge The cloud mobility and leaves how does AI strengthen information security in a commentary on data?
SecurityInterestingly enough, Edge AI has the potential to improve security by keeping sensitive data local and reducing the frequency of transmitting such data over often insecure networks.
So which businesses stand to gain from cloud-edge AI?
Because edge AI processes and acts on data in real time with low latency, the technology is especially useful for industries such as healthcare, manufacturing, automotive ad smart cities.
What are the primary reasons that make deploying edge AI solutions hard?
Challenges include data security, ensuring network reliability and the complexity of deployment and management edge AI infrastructure.
So, how does 5G technology changes the integration of edge AI with cloud computing?
Edge AI helps to make the real-time processing more efficient 5G technology brings faster data transfer rates with low latency which again enhances performance and capabilities of edge AI applications.